DATA Processing
Contents
DATA ProcessingΒΆ
The purpose of this Notebook is to perform the data processing steps for each sample:
DP1 : Substraction
DP2 : Gaussian fitting
One aim is to make this Notebook working within an html page and interactive using ipython widget
WorkflowΒΆ
#
To create (drag and drop image when produced)
LibrariesΒΆ
import numpy
import math
import matplotlib
import matplotlib.pyplot as plt
from matplotlib import cm
import pandas as pd
from glob import glob
from functools import reduce
import ipywidgets as widgets
import matplotlib.gridspec as gridspec
from ipywidgets import interact
import re
from cycler import cycler
import seaborn as sns
import matplotlib.gridspec as gridspec
import pylab
from lmfit.models import ExponentialModel, GaussianModel
%matplotlib
Using matplotlib backend: Qt5Agg
0 Input parametersΒΆ
Not use (but here just in case)
Take time to describe method used with respect to previous Notebook (Improvement - Emphase on python teaching)
Sample typeΒΆ
# 0.1 Date
date = "2020_09_16"
# 0.2 XP
# Can take value XP_1-1, XP_1-2
XP = "XP_1-1"
# 0.3 Sample type
# Can take value ASW, C2H6, C2H6_ASW
spl = "ASW"
Sample annexΒΆ
For quick sample comparison (max 3 sample named 2-3-4)
date2 = "2020_09_28"
date3 = "2020_09_17"
date4 = "2020_09_21"
\(\color{green}{\text{DP1 : Sample Checking}}\) ΒΆ
1.1 DATA ImportΒΆ
The Data processing routine is performed per sample.
A date is set as input
from the previous data analysis, summarized in the following document below, we have identified 4 temeperature ranges where the sample undergo different physical processes.
XP1_Plan
So the purpose here is to chop the data with respect to those ranges before to perform the Substraction
Experimental ranges (in T):
Range 1: 20 - 100K
Range 2: 100 to 130K
Range 3: 130K - 140K
Range 4: Beyond 140K
I want to extract the temperature ramp info from XP Ramp:
1.1.1 XP-RampΒΆ
XP_Ramp_df = pd.read_csv('D:\DATA-Processing\PAC\XP_list_test.csv')
XP_Ramp_df_I = XP_Ramp_df.set_index('Date')
#XP_Ramp_df_I.head(5)
1.1.2 DR1, DR2, DR3ΒΆ
DR1 (Date)ΒΆ
DR1_Allscan_df = pd.read_csv("D:\DATA-Processing\PAC\{}/Samples/{}/Data/DR/DR1_{}_All-scans.csv".format(XP,date,date))
DR1_Allscan_df = DR1_Allscan_df.T.iloc[1:].T
#DR1_Allscan_df
DR1 (Date Annex)ΒΆ
DR1_Allscan_df_2 = pd.read_csv("D:\DATA-Processing\PAC\{}/Samples/{}/Data/DR/DR1_{}_All-scans.csv".format(XP,date2,date2))
DR1_Allscan_df_3 = pd.read_csv("D:\DATA-Processing\PAC\{}/Samples/{}/Data/DR/DR1_{}_All-scans.csv".format(XP,date3,date3))
DR1_Allscan_df_4 = pd.read_csv("D:\DATA-Processing\PAC\{}/Samples/{}/Data/DR/DR1_{}_All-scans.csv".format(XP,date4,date4))
DR1_Allscan_df_2 = DR1_Allscan_df_2.T.iloc[1:].T
DR1_Allscan_df_3 = DR1_Allscan_df_3.T.iloc[1:].T
DR1_Allscan_df_4 = DR1_Allscan_df_4.T.iloc[1:].T
#DR1_Allscan_df_2
DR2 (Date)ΒΆ
DR2_Allscan_df = pd.read_csv('D:\DATA-Processing\PAC\{}/Samples/{}/Data/DR/DR2_{}_All-scans.csv'.format(XP, date, date))
DR2_Allscan_df = DR2_Allscan_df.T.iloc[1:].T
#DR2_Allscan_df
DR2 (Date Annex)ΒΆ
DR2_Allscan_df_2 = pd.read_csv('D:\DATA-Processing\PAC\{}/Samples/{}/Data/DR/DR2_{}_All-scans.csv'.format(XP, date2, date2))
DR2_Allscan_df_3 = pd.read_csv('D:\DATA-Processing\PAC\{}/Samples/{}/Data/DR/DR2_{}_All-scans.csv'.format(XP, date3, date3))
DR2_Allscan_df_4 = pd.read_csv('D:\DATA-Processing\PAC\{}/Samples/{}/Data/DR/DR2_{}_All-scans.csv'.format(XP, date4, date4))
DR2_Allscan_df_2 = DR2_Allscan_df_2.T.iloc[1:].T
DR2_Allscan_df_3 = DR2_Allscan_df_3.T.iloc[1:].T
DR2_Allscan_df_4 = DR2_Allscan_df_4.T.iloc[1:].T
#DR2_Allscan_df_2
DR3 (Date)ΒΆ
DR3_Allscan_df = pd.read_csv("D:\DATA-Processing\PAC\{}/Samples/{}/Data/DR/DR3_{}_A.csv".format(XP,date,date))
DR3_Allscan_df = DR3_Allscan_df.T.iloc[1:].T
#DR3_Allscan_df
DR3 (Date Annex)ΒΆ
DR3_Allscan_df_2 = pd.read_csv("D:\DATA-Processing\PAC\{}/Samples/{}/Data/DR/DR3_{}_A.csv".format(XP,date2,date2))
DR3_Allscan_df_3 = pd.read_csv("D:\DATA-Processing\PAC\{}/Samples/{}/Data/DR/DR3_{}_A.csv".format(XP,date3,date3))
DR3_Allscan_df_4 = pd.read_csv("D:\DATA-Processing\PAC\{}/Samples/{}/Data/DR/DR3_{}_A.csv".format(XP,date4,date4))
DR3_Allscan_df_2 = DR3_Allscan_df_2.T.iloc[1:].T
DR3_Allscan_df_3 = DR3_Allscan_df_3.T.iloc[1:].T
DR3_Allscan_df_4 = DR3_Allscan_df_4.T.iloc[1:].T
1.3 Data_annexΒΆ
1.2.1 ImportΒΆ
#Date
data_anex_df = pd.read_csv("D:\DATA-Processing\PAC\{}/Samples/{}/Data/DR/{}_data_annex.csv".format(XP,date,date))
#Date Annex
data_anex_df_2 = pd.read_csv("D:\DATA-Processing\PAC\{}/Samples/{}/Data/DR/{}_data_annex.csv".format(XP,date2,date2))
data_anex_df_3 = pd.read_csv("D:\DATA-Processing\PAC\{}/Samples/{}/Data/DR/{}_data_annex.csv".format(XP,date3,date3))
data_anex_df_4 = pd.read_csv("D:\DATA-Processing\PAC\{}/Samples/{}/Data/DR/{}_data_annex.csv".format(XP,date4,date4))
data_anex_df
Name | min1 | index1 | min2 | index2 | min3 | index3 | min4 | index4 | min5 | ... | maxA0w | maxBi | maxB | maxBw | maxCi | maxC | maxCw | Int_A | Int_C | Int_N_A | |
---|---|---|---|---|---|---|---|---|---|---|---|---|---|---|---|---|---|---|---|---|---|
0 | ASW_2020_09_16_1 | -0.018844 | 6636 | -0.008464 | 4235 | -0.008369 | 4139 | -0.001418 | 2403 | -0.000786 | ... | 3268.321 | 2945 | 0.009284 | 2219.700 | 1773 | 0.023557 | 1654.651 | 95.913079 | 6.952239 | 100.000000 |
1 | ASW_2020_09_16_2 | -0.017376 | 6636 | -0.006804 | 4235 | -0.006714 | 4139 | 0.000216 | 2432 | 0.000863 | ... | 3264.464 | 2945 | 0.009251 | 2219.700 | 1771 | 0.023602 | 1653.686 | 96.271574 | 7.064048 | 100.373772 |
2 | ASW_2020_09_16_3 | -0.015208 | 6636 | -0.005247 | 4197 | -0.005165 | 4130 | 0.001310 | 2413 | 0.001796 | ... | 3264.464 | 2947 | 0.010343 | 2220.665 | 1754 | 0.024362 | 1645.490 | 98.372535 | 7.669780 | 102.564255 |
3 | ASW_2020_09_16_4 | -0.014749 | 6631 | -0.004931 | 4197 | -0.004867 | 4130 | 0.001626 | 2413 | 0.002152 | ... | 3264.464 | 2947 | 0.010512 | 2220.665 | 1754 | 0.024289 | 1645.490 | 98.949916 | 7.710412 | 103.166239 |
4 | ASW_2020_09_16_5 | -0.014274 | 6620 | -0.004424 | 4245 | -0.004362 | 4130 | 0.002212 | 2413 | 0.002702 | ... | 3264.464 | 2947 | 0.010562 | 2220.665 | 1754 | 0.024293 | 1645.490 | 99.128959 | 7.740047 | 103.352911 |
5 | ASW_2020_09_16_6 | -0.013616 | 6636 | -0.003845 | 4235 | -0.003754 | 4139 | 0.003361 | 2444 | 0.003725 | ... | 3255.303 | 2949 | 0.010078 | 2221.629 | 1756 | 0.023139 | 1646.454 | 102.427355 | 8.047927 | 106.791854 |
6 | ASW_2020_09_16_7 | -0.013178 | 6632 | -0.003492 | 4235 | -0.003383 | 4139 | 0.003972 | 2425 | 0.004340 | ... | 3255.303 | 2951 | 0.010252 | 2222.593 | 1756 | 0.022505 | 1646.454 | 103.570509 | 8.057326 | 107.983719 |
7 | ASW_2020_09_16_8 | -0.012907 | 6632 | -0.003190 | 4235 | -0.003086 | 4139 | 0.004323 | 2406 | 0.004697 | ... | 3255.303 | 2951 | 0.010345 | 2222.593 | 1756 | 0.022478 | 1646.454 | 103.920588 | 8.069566 | 108.348714 |
8 | ASW_2020_09_16_9 | -0.013285 | 6636 | -0.002969 | 4245 | -0.002883 | 4139 | 0.004149 | 2415 | 0.004730 | ... | 3251.446 | 2959 | 0.011390 | 2226.450 | 1754 | 0.022548 | 1645.490 | 106.316388 | 8.023931 | 110.846602 |
9 | ASW_2020_09_16_10 | -0.013301 | 6636 | -0.002779 | 4216 | -0.002666 | 4139 | 0.004334 | 2367 | 0.004952 | ... | 3251.446 | 2959 | 0.011787 | 2226.450 | 1754 | 0.022958 | 1645.490 | 107.623907 | 8.026164 | 112.209835 |
10 | ASW_2020_09_16_11 | -0.013018 | 6636 | -0.002502 | 4197 | -0.002379 | 4120 | 0.004644 | 2367 | 0.005298 | ... | 3250.482 | 2959 | 0.011822 | 2226.450 | 1754 | 0.022904 | 1645.490 | 107.980853 | 8.037065 | 112.581991 |
11 | ASW_2020_09_16_12 | -0.012113 | 6636 | -0.002024 | 4149 | -0.001986 | 4130 | 0.005265 | 2415 | 0.005871 | ... | 3251.446 | 2959 | 0.011693 | 2226.450 | 1754 | 0.020700 | 1645.490 | 108.386906 | 8.033410 | 113.005345 |
12 | ASW_2020_09_16_13 | -0.011900 | 6636 | -0.001360 | 4151 | -0.001341 | 4132 | 0.005725 | 2367 | 0.006299 | ... | 3250.482 | 2980 | 0.012006 | 2236.575 | 1771 | 0.021517 | 1653.686 | 109.276611 | 8.503723 | 113.932962 |
13 | ASW_2020_09_16_14 | -0.011673 | 6636 | -0.001157 | 4151 | -0.001115 | 4130 | 0.005912 | 2415 | 0.006504 | ... | 3250.482 | 2959 | 0.011885 | 2226.450 | 1771 | 0.021516 | 1653.686 | 109.502264 | 8.477687 | 114.168230 |
14 | ASW_2020_09_16_15 | -0.011645 | 6636 | -0.001251 | 4149 | -0.001226 | 4139 | 0.006022 | 2415 | 0.006723 | ... | 3250.482 | 2966 | 0.011713 | 2229.825 | 1699 | 0.020339 | 1618.973 | 109.378492 | 8.007280 | 114.039184 |
15 | ASW_2020_09_16_16 | -0.011338 | 6636 | -0.000905 | 4168 | -0.000901 | 4139 | 0.006207 | 2415 | 0.006852 | ... | 3247.107 | 2964 | 0.012078 | 2228.861 | 1769 | 0.020189 | 1652.722 | 109.838625 | 8.093852 | 114.518923 |
16 | ASW_2020_09_16_17 | -0.011244 | 6636 | -0.000821 | 4149 | -0.000807 | 4139 | 0.006330 | 2415 | 0.006958 | ... | 3247.107 | 2964 | 0.012166 | 2228.861 | 1769 | 0.020213 | 1652.722 | 110.041893 | 8.142568 | 114.730852 |
17 | ASW_2020_09_16_18 | -0.011332 | 6636 | -0.000989 | 4149 | -0.001025 | 4130 | 0.006762 | 2480 | 0.007738 | ... | 3246.625 | 2957 | 0.011663 | 2225.486 | 1754 | 0.021377 | 1645.490 | 109.763420 | 7.476483 | 114.440514 |
18 | ASW_2020_09_16_19 | -0.010872 | 6636 | -0.000882 | 4158 | -0.000914 | 4130 | 0.006530 | 2480 | 0.008113 | ... | 3246.625 | 2978 | 0.011855 | 2235.610 | 1697 | 0.021481 | 1618.009 | 109.666245 | 7.569996 | 114.339198 |
19 | ASW_2020_09_16_20 | -0.010809 | 6636 | -0.000910 | 4149 | -0.000942 | 4130 | 0.006549 | 2480 | 0.008185 | ... | 3246.143 | 2978 | 0.011966 | 2235.610 | 1697 | 0.021457 | 1618.009 | 109.738473 | 7.560006 | 114.414504 |
20 | ASW_2020_09_16_21 | -0.011159 | 6636 | -0.001222 | 4151 | -0.001287 | 4103 | 0.006266 | 2415 | 0.007035 | ... | 3242.286 | 2966 | 0.012110 | 2229.825 | 1699 | 0.020884 | 1618.973 | 109.665610 | 8.124397 | 114.338537 |
21 | ASW_2020_09_16_22 | -0.010915 | 6630 | -0.001349 | 4151 | -0.001461 | 4111 | 0.006305 | 2444 | 0.007058 | ... | 3232.161 | 2976 | 0.012184 | 2234.646 | 1535 | 0.020529 | 1539.905 | 109.891203 | 8.369954 | 114.573742 |
22 | ASW_2020_09_16_23 | -0.010836 | 6630 | -0.001540 | 4151 | -0.001650 | 4084 | 0.006335 | 2396 | 0.007176 | ... | 3228.304 | 2976 | 0.012485 | 2234.646 | 1535 | 0.020793 | 1539.905 | 110.395286 | 8.440394 | 115.099305 |
23 | ASW_2020_09_16_24 | -0.014164 | 6636 | -0.006225 | 4149 | -0.006255 | 4101 | 0.003633 | 2444 | 0.004862 | ... | 3228.304 | 2976 | 0.011491 | 2234.646 | 1697 | 0.019248 | 1618.009 | 108.090993 | 7.265889 | 112.696823 |
24 | ASW_2020_09_16_25 | -0.013780 | 6636 | -0.008598 | 4151 | -0.008599 | 4122 | 0.001659 | 2484 | 0.002442 | ... | 3228.304 | 2978 | 0.008953 | 2235.610 | 1535 | 0.016275 | 1539.905 | 83.840839 | 5.785232 | 87.413354 |
25 | ASW_2020_09_16_26 | 0.002459 | 6636 | 0.004937 | 4151 | 0.004895 | 4053 | 0.006344 | 2329 | 0.006488 | ... | 3227.822 | 2978 | 0.004050 | 2235.610 | 1535 | 0.009392 | 1539.905 | 27.229288 | 2.969525 | 28.389547 |
26 | ASW_2020_09_16_27 | 0.007303 | 6636 | 0.007753 | 4533 | 0.008098 | 3959 | 0.007518 | 2300 | 0.007247 | ... | 3228.304 | 2295 | 0.001530 | 1906.319 | 1495 | 0.004807 | 1520.620 | 3.170572 | 1.399266 | 3.305672 |
27 | ASW_2020_09_16_28 | 0.007890 | 6332 | 0.008135 | 4545 | 0.008503 | 4139 | 0.008035 | 2480 | 0.007282 | ... | 3242.286 | 2698 | 0.001674 | 2100.615 | 1495 | 0.006589 | 1520.620 | 2.966754 | 1.841489 | 3.093169 |
28 | ASW_2020_09_16_29 | 0.008264 | 6332 | 0.008545 | 4563 | 0.008896 | 4139 | 0.008449 | 2480 | 0.007719 | ... | 3242.286 | 2698 | 0.001720 | 2100.615 | 1495 | 0.006580 | 1520.620 | 3.023007 | 1.840784 | 3.151819 |
29 | ASW_2020_09_16_30 | 0.008619 | 6331 | 0.008641 | 4562 | 0.009109 | 4084 | 0.008596 | 2480 | 0.008381 | ... | 3228.304 | 2602 | 0.001573 | 2054.332 | 1574 | 0.004599 | 1558.708 | 3.025809 | 1.338555 | 3.154741 |
30 | ASW_2020_09_16_31 | 0.008318 | 6636 | 0.008889 | 4543 | 0.009277 | 3959 | 0.008351 | 2461 | 0.008204 | ... | 3223.965 | 2437 | 0.002416 | 1974.781 | 1495 | 0.006633 | 1520.620 | 3.256800 | 1.924223 | 3.395574 |
31 rows Γ 31 columns
#data_anex_df = data_anex_df.T.iloc[1:].T
#data_anex_df_2 = data_anex_df_2.T.iloc[1:].T
#data_anex_df_3 = data_anex_df_3.T.iloc[1:].T
#data_anex_df_4 = data_anex_df_4.T.iloc[1:].T
data_anex_df_2
Name | min1 | index1 | min2 | index2 | min3 | index3 | min4 | index4 | min5 | ... | maxA0w | maxBi | maxB | maxBw | maxCi | maxC | maxCw | Int_A | Int_C | Int_N_A | |
---|---|---|---|---|---|---|---|---|---|---|---|---|---|---|---|---|---|---|---|---|---|
0 | ASW_2020_09_28_1 | -0.026504 | 6620 | -0.024226 | 4401 | -0.022407 | 4134 | -0.009105 | 2458 | -0.007995 | ... | 3272.178 | 2923 | 0.009211 | 2209.094 | 1780 | 0.022529 | 1658.026 | 86.558093 | 6.591012 | 100.000000 |
1 | ASW_2020_09_28_2 | -0.025427 | 6636 | -0.022822 | 4372 | -0.021184 | 4144 | -0.007454 | 2477 | -0.006254 | ... | 3276.035 | 2942 | 0.007386 | 2218.254 | 1771 | 0.019989 | 1653.686 | 86.043652 | 5.563222 | 99.405669 |
2 | ASW_2020_09_28_3 | -0.024675 | 6636 | -0.021948 | 4401 | -0.020278 | 4142 | -0.006614 | 2477 | -0.005435 | ... | 3276.035 | 2942 | 0.007681 | 2218.254 | 1771 | 0.020173 | 1653.686 | 86.876902 | 5.599657 | 100.368318 |
3 | ASW_2020_09_28_4 | -0.024353 | 6632 | -0.021656 | 4393 | -0.019961 | 4142 | -0.006287 | 2477 | -0.005064 | ... | 3271.213 | 2923 | 0.007746 | 2209.094 | 1771 | 0.020165 | 1653.686 | 87.117734 | 5.607032 | 100.646549 |
4 | ASW_2020_09_28_5 | -0.024163 | 6632 | -0.021448 | 4401 | -0.019691 | 4134 | -0.005975 | 2458 | -0.004768 | ... | 3276.035 | 2942 | 0.007936 | 2218.254 | 1771 | 0.020096 | 1653.686 | 87.298165 | 5.540918 | 100.855000 |
... | ... | ... | ... | ... | ... | ... | ... | ... | ... | ... | ... | ... | ... | ... | ... | ... | ... | ... | ... | ... | ... |
75 | ASW_2020_09_28_76 | 0.033360 | 6633 | 0.035411 | 4154 | 0.035383 | 4135 | 0.040743 | 2479 | 0.041975 | ... | 3226.858 | 2990 | 0.005160 | 2241.396 | 1675 | 0.008315 | 1607.402 | 40.624815 | 2.506664 | 46.933584 |
76 | ASW_2020_09_28_77 | 0.039784 | 6633 | 0.040950 | 4154 | 0.040848 | 4077 | 0.043054 | 2479 | 0.043847 | ... | 3225.894 | 3040 | 0.003348 | 2265.502 | 1675 | 0.006200 | 1607.402 | 22.869835 | 1.940948 | 26.421371 |
77 | ASW_2020_09_28_78 | 0.043527 | 6335 | 0.043778 | 4163 | 0.043654 | 3962 | 0.043991 | 2345 | 0.043920 | ... | 3224.929 | 2300 | 0.002073 | 1908.730 | 947 | 0.005038 | 1256.416 | 5.198979 | 2.124355 | 6.006347 |
78 | ASW_2020_09_28_79 | 0.043639 | 6638 | 0.043704 | 4557 | 0.044042 | 3943 | 0.044228 | 2345 | 0.044163 | ... | 3224.929 | 2321 | 0.002171 | 1918.855 | 947 | 0.005697 | 1256.416 | 3.493992 | 2.447063 | 4.036586 |
79 | ASW_2020_09_28_80 | 0.016138 | 6633 | 0.020018 | 4211 | 0.020286 | 4136 | 0.033108 | 2489 | 0.034497 | ... | 3248.072 | 3037 | 0.011578 | 2264.056 | 1698 | 0.018059 | 1618.491 | 102.387413 | 7.200944 | 118.287510 |
80 rows Γ 31 columns
Purpose of this section is to perform a deep analysis of the sample with respect to the temperature ramp as for example,
plots various scans at different temperature, identify various range of interest.
perform linear regression of desorption data
average scans when isotherm ?
1.2 DATA CleaningΒΆ
1.2.1 Annealing scan supression and averaging of the isotherms ?ΒΆ
1.2.2 Error AnalysisΒΆ
After having avaeraged the scan previously, the purpose of this section is to identify the potential sources of errors and to generate error bars for the data accordingly
1.2 DATA CompareΒΆ
1.2.1 MaxAΒΆ
Prepare two dictionnariesΒΆ
Dict 1ΒΆ
16-09
i = [0,1,4,7,10,13,16,19,22,25]
j = ["Dep","20","40","60","80","100","120","130","140","160"]
Td = dict(zip(j, i))
nscanTd = len(list(data_anex_df.index.values.tolist()))
print(nscanTd)
print(Td)
31
{'Dep': 0, '20': 1, '40': 4, '60': 7, '80': 10, '100': 13, '120': 16, '130': 19, '140': 22, '160': 25}
Dict 2ΒΆ
28-09
i = [0,1,8,16,24,32,40,52,60,79]
j = ["Dep","20","40","60","80","100","120","130","140","160"]
T2d = dict(zip(j, i))
nscanT2d = len(list(data_anex_df_2.index.values.tolist()))
print(nscanT2d)
print(T2d)
80
{'Dep': 0, '20': 1, '40': 8, '60': 16, '80': 24, '100': 32, '120': 40, '130': 52, '140': 60, '160': 79}
PlotΒΆ
Peak frequency shiftΒΆ
fig= plt.figure(figsize=(10,8))
for (k,v), (k2,v2) in zip(Td.items(), T2d.items()):
spl1, = plt.plot(k, data_anex_df.iloc[v,17], '+', mew=3, ms=12, c=cm.jet(v/nscanTd), label= "16_09")
spl2, = plt.plot(k2, data_anex_df_2.iloc[v2,17], 'o', mew=3, ms=10, c=cm.jet(v2/nscanT2d), label= "28_09")
spl3, = plt.plot(k, data_anex_df_3.iloc[v,17], '.', mew=3, ms=12, c=cm.jet(v/nscanTd), label= "17_09")
spl4, = plt.plot(k, data_anex_df_4.iloc[v,17], '*', mew=3, ms=12, c=cm.jet(v/nscanTd), label= "21_09")
plt.title('DR2 OH stretch Peak frequency (sample comparison)')
#plt.axis([3210,3270])
plt.xlabel('Temperature (K)').set_fontsize(13)
plt.ylabel('Peak frequency (cm-1)').set_fontsize(13)
#ax = fig.gca()
#plt.grid()
#plt.legend()
plt.legend([spl1, spl2, spl3, spl4], ["16_09", "28_09", "17_09", "21_09"],bbox_to_anchor =(1.25, 1),)
#plt.savefig('D:\DATA-Processing\PAC\{}/Compare/DR2_{}_{}_PeakA-frequency.png'.format(XP, date, date2))
plt.show()
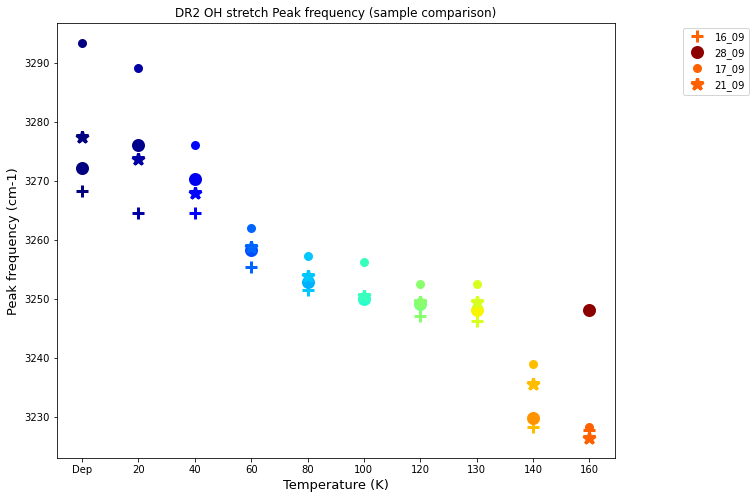
Integration from normalized dataΒΆ
fig= plt.figure(figsize=(10,8))
for (k,v), (k2,v2) in zip(Td.items(), T2d.items()):
spl1, = plt.plot(k, data_anex_df.iloc[v,30], '+', mew=3, ms=12, c=cm.jet(v/nscanTd), label= "16_09")
spl2, = plt.plot(k2, data_anex_df_2.iloc[v2,30], 'o', mew=3, ms=10, c=cm.jet(v2/nscanT2d), label= "28_09")
spl3, = plt.plot(k, data_anex_df_3.iloc[v,30], '.', mew=3, ms=12, c=cm.jet(v/nscanTd), label= "17_09")
spl4, = plt.plot(k, data_anex_df_4.iloc[v,30], '*', mew=3, ms=12, c=cm.jet(v/nscanTd), label= "21_09")
plt.title('DR2 OH stretch Integration value (sample comparison)')
#plt.axis([3210,3270])
plt.xlabel('Temperature (K)').set_fontsize(13)
plt.ylabel('Integration (AU)').set_fontsize(13)
#ax = fig.gca()
#plt.grid()
#plt.legend()
plt.legend([spl1, spl2, spl3, spl4], ["16_09", "28_09", "17_09", "21_09"])
#plt.savefig('D:\DATA-Processing\PAC\{}/Compare/DR2_{}_{}_PeakA-frequency.png'.format(XP, date, date2))
plt.show()
1.3 Data choppingΒΆ
1.3.1 Temperature list splittingΒΆ
Temp = XP_Ramp_df_I.columns[1:].values.tolist()
Temp
['20K ',
'30K',
'40K',
'50K',
'60K',
'70K',
'80K',
'90K',
'100K',
'110K',
'120K',
'125K',
'130K',
'132K',
'134K',
'135K',
'136K',
'137K',
'138K',
'140K',
'142K',
'145K',
'150K',
'155K',
'160K',
'180K',
'200K']
iterate through temp and recover scan number value for T in range 1,2,3,4
T1 = Temp[0:9]
T2 = Temp[8:13]
T3 = Temp[12:20]
T4 = Temp[19:26]
Sanity CheckΒΆ
T2
['100K', '110K', '120K', '125K', '130K']
1.1.3 List of sample names per rangeΒΆ
Range 1ΒΆ
z = []
value_1 = []
file_number1 = []
for y in T1:
value = XP_Ramp_df_I.loc[XP_Ramp_df_I.index == date, y].values[0]
if pd.notnull(value):
value_1 = re.findall(r"[-+]?\d*\.\d+|\d+", value)
for items in value_1:
to_plot = str('{}_{}_{}'.format(spl, date, items))
z.append({
'Name' : str(to_plot),
'Temp' : y,
'Date': date,
})
file_number1.append(int(items))
dat1= pd.DataFrame(z)
R1_df = dat1.set_index('Name')
z
[{'Name': 'ASW_2021_05_24_1', 'Temp': '20K ', 'Date': '2021_05_24'},
{'Name': 'ASW_2021_05_24_2', 'Temp': '20K ', 'Date': '2021_05_24'},
{'Name': 'ASW_2021_05_24_3', 'Temp': '20K ', 'Date': '2021_05_24'},
{'Name': 'ASW_2021_05_24_4', 'Temp': '70K', 'Date': '2021_05_24'},
{'Name': 'ASW_2021_05_24_5', 'Temp': '70K', 'Date': '2021_05_24'},
{'Name': 'ASW_2021_05_24_6', 'Temp': '70K', 'Date': '2021_05_24'},
{'Name': 'ASW_2021_05_24_7', 'Temp': '70K', 'Date': '2021_05_24'}]
R1_df
Temp | Date | |
---|---|---|
Name | ||
ASW_2021_05_24_1 | 20K | 2021_05_24 |
ASW_2021_05_24_2 | 20K | 2021_05_24 |
ASW_2021_05_24_3 | 20K | 2021_05_24 |
ASW_2021_05_24_4 | 70K | 2021_05_24 |
ASW_2021_05_24_5 | 70K | 2021_05_24 |
ASW_2021_05_24_6 | 70K | 2021_05_24 |
ASW_2021_05_24_7 | 70K | 2021_05_24 |
Range 2ΒΆ
z = []
value_1 = []
file_number2 = []
for y in T2:
value = XP_Ramp_df_I.loc[XP_Ramp_df_I.index == date, y].values[0]
if pd.notnull(value):
value_1 = re.findall(r"[-+]?\d*\.\d+|\d+", value)
for items in value_1:
to_plot = str('{}_{}_{}'.format(spl, date, items))
z.append({
'Name' : str(to_plot),
'Temp' : y,
'Date': date,
})
file_number2.append(int(items))
dat2= pd.DataFrame(z)
R2_df = dat2.set_index('Name')
---------------------------------------------------------------------------
KeyError Traceback (most recent call last)
<ipython-input-21-5bf50306e56a> in <module>
24
25 dat2= pd.DataFrame(z)
---> 26 R2_df = dat2.set_index('Name')
~\Anaconda3\lib\site-packages\pandas\core\frame.py in set_index(self, keys, drop, append, inplace, verify_integrity)
4301
4302 if missing:
-> 4303 raise KeyError(f"None of {missing} are in the columns")
4304
4305 if inplace:
KeyError: "None of ['Name'] are in the columns"
R2_df
Range 3ΒΆ
z = []
value_1 = []
file_number3 = []
for y in T3:
value = XP_Ramp_df_I.loc[XP_Ramp_df_I.index == date, y].values[0]
if pd.notnull(value):
value_1 = re.findall(r"[-+]?\d*\.\d+|\d+", value)
for items in value_1:
to_plot = str('{}_{}_{}'.format(spl, date, items))
z.append({
'Name' : str(to_plot),
'Temp' : y,
'Date': date,
})
file_number3.append(int(items))
dat3= pd.DataFrame(z)
R3_df = dat3.set_index('Name')
R3_df
Temp | Date | |
---|---|---|
Name | ||
ASW_2021_05_24_8 | 135K | 2021_05_24 |
ASW_2021_05_24_9 | 135K | 2021_05_24 |
ASW_2021_05_24_10 | 135K | 2021_05_24 |
ASW_2021_05_24_11 | 135K | 2021_05_24 |
ASW_2021_05_24_12 | 135K | 2021_05_24 |
... | ... | ... |
ASW_2021_05_24_414 | 140K | 2021_05_24 |
ASW_2021_05_24_415 | 140K | 2021_05_24 |
ASW_2021_05_24_416 | 140K | 2021_05_24 |
ASW_2021_05_24_417 | 140K | 2021_05_24 |
ASW_2021_05_24_418 | 140K | 2021_05_24 |
411 rows Γ 2 columns
Range 4ΒΆ
z = []
value_1 = []
file_number4 = []
for y in T4:
value = XP_Ramp_df_I.loc[XP_Ramp_df_I.index == date, y].values[0]
if pd.notnull(value):
value_1 = re.findall(r"[-+]?\d*\.\d+|\d+", value)
for items in value_1:
to_plot = str('{}_{}_{}'.format(spl, date, items))
z.append({
'Name' : str(to_plot),
'Temp' : y,
'Date': date,
})
file_number4.append(int(items))
dat4= pd.DataFrame(z)
R4_df = dat4.set_index('Name')
R4_df
Temp | Date | |
---|---|---|
Name | ||
ASW_2021_05_24_393 | 140K | 2021_05_24 |
ASW_2021_05_24_394 | 140K | 2021_05_24 |
ASW_2021_05_24_395 | 140K | 2021_05_24 |
ASW_2021_05_24_396 | 140K | 2021_05_24 |
ASW_2021_05_24_397 | 140K | 2021_05_24 |
... | ... | ... |
ASW_2021_05_24_495 | 145K | 2021_05_24 |
ASW_2021_05_24_496 | 145K | 2021_05_24 |
ASW_2021_05_24_497 | 145K | 2021_05_24 |
ASW_2021_05_24_498 | 145K | 2021_05_24 |
ASW_2021_05_24_499 | 145K | 2021_05_24 |
107 rows Γ 2 columns
1.4 PlottingΒΆ
Plot 4 subplot in square formating for all 4 T range
1.4.1 T rangesΒΆ
GridspecΒΆ
gs = gridspec.GridSpec(16, 16,hspace=0.8,wspace=0.5)
fig = plt.figure(figsize=(16,16))
fig.suptitle('20K 10-7 All samples')
ax1 = fig.add_subplot(gs[0:8, 0:8]) # row 0, col 0
nscan = len(list(R1_df['Date'].values.tolist()))
j = 1
for i, j in zip(dat1['Name'], range(1,nscan)):
# for iscan in range(1,nscan):
#DR2
#x = DR2_Allscans_full.Wavenumber
#y = DR2_Allscans_full['{}'.format(i)]
x = DR3_Allscan_df.Wavenumber
y = DR3_Allscan_df['{}'.format(i)]
plt.plot(x,y, label="{}_{}".format(str(R1_df.loc[i]['Date']), str(R1_df.loc[i]['Temp'])), c=cm.jet(j/nscan))
j=+1
ax1.set_title('Full range')
ax1.axis([4000, 2800, 0, 0.5])
plt.legend()
ax2 = fig.add_subplot(gs[0:8, 8:16]) # row 0, col 0
nscan = len(list(R2_df['Date'].values.tolist()))
j = 1
for i, j in zip(dat2['Name'], range(1,nscan)):
# for iscan in range(1,nscan):
#DR2
#x = DR2_Allscans_full.Wavenumber
#y = DR2_Allscans_full['{}'.format(i)]
x = DR3_Allscan_df.Wavenumber
y = DR3_Allscan_df['{}'.format(i)]
plt.plot(x,y, label="{}_{}".format(str(R2_df.loc[i]['Date']), str(R2_df.loc[i]['Temp'])), c=cm.jet(j/nscan))
j=+1
ax2.set_title('Normalized zoom')
ax2.margins(2, 2)
ax2.axis([4000, 2800, 0, 0.5])
plt.legend()
ax3 = fig.add_subplot(gs[8:16, 0:8]) # row 0, col 0
nscan = len(list(R3_df['Date'].values.tolist()))
j = 1
for i, j in zip(dat3['Name'], range(1,nscan)):
# for iscan in range(1,nscan):
#DR2
#x = DR2_Allscans_full.Wavenumber
#y = DR2_Allscans_full['{}'.format(i)]
x = DR3_Allscan_df.Wavenumber
y = DR3_Allscan_df['{}'.format(i)]
plt.plot(x,y, label="{}_{}".format(str(R3_df.loc[i]['Date']), str(R3_df.loc[i]['Temp'])), c=cm.jet(j/nscan))
j=+1
ax3.set_title('BC zoomed')
ax3.axis([4000, 2800, 0, 0.5])
plt.legend()
ax4 = fig.add_subplot(gs[8:16, 8:16]) # row 0, col 0
nscan = len(list(R4_df['Date'].values.tolist()))
j = 1
for i, j in zip(dat4['Name'], range(1,nscan)):
# for iscan in range(1,nscan):
#DR2
#x = DR2_Allscans_full.Wavenumber
#y = DR2_Allscans_full['{}'.format(i)]
x = DR3_Allscan_df.Wavenumber
y = DR3_Allscan_df['{}'.format(i)]
plt.plot(x,y, label="{}_{}".format(str(R4_df.loc[i]['Date']), str(R4_df.loc[i]['Temp'])), c=cm.jet(j/nscan))
j=+1
ax4.set_title('BC zoomed')
ax4.axis([4000, 2800, 0, 0.5])
plt.legend()
plt.show()
---------------------------------------------------------------------------
NameError Traceback (most recent call last)
<ipython-input-26-d01ae63ef9a4> in <module>
30
31 ax2 = fig.add_subplot(gs[0:8, 8:16]) # row 0, col 0
---> 32 nscan = len(list(R2_df['Date'].values.tolist()))
33
34
NameError: name 'R2_df' is not defined
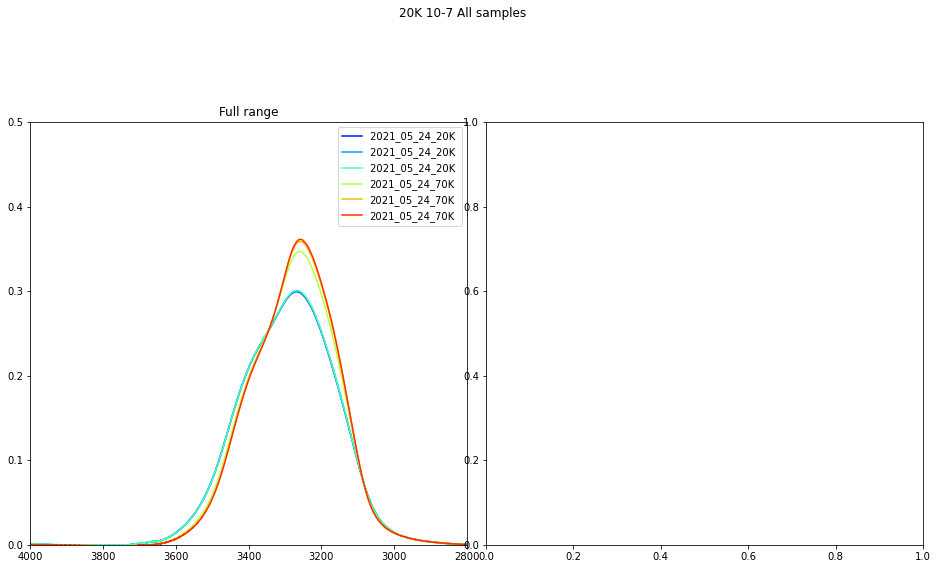
insert condition to supress first scan (ramp) Implement maxA Increase spacing between plots
1.6 Scan Averaging and error analysis ?ΒΆ
Supress the first scan and average the remaining scan
Have a though about error bars
1.7 Data processingΒΆ
1.7.1 Linear regression of desorptionΒΆ
This is where some fitting options needs to be implemented ie:
linear regression for desorption data
Polynomial for isotherms at 130, 135K ?
1.7.2 polynomial fitting of isotherm at 130KΒΆ
Goal,
\(\color{green}{\text{DP2 : Substraction scan}}\) ΒΆ
use DR2 data
Purpose here is to obtain 4 different substraction spectra for each of the presestablished range (use hot-warm color to plot)
2.1 Data Sanity CheckΒΆ
DR2_Allscan_df
Wavenumber | ASW_2021_05_24_1 | ASW_2021_05_24_2 | ASW_2021_05_24_3 | ASW_2021_05_24_4 | ASW_2021_05_24_5 | ASW_2021_05_24_6 | ASW_2021_05_24_7 | ASW_2021_05_24_8 | ASW_2021_05_24_9 | ... | ASW_2021_05_24_483 | ASW_2021_05_24_484 | ASW_2021_05_24_485 | ASW_2021_05_24_486 | ASW_2021_05_24_487 | ASW_2021_05_24_488 | ASW_2021_05_24_489 | ASW_2021_05_24_490 | ASW_2021_05_24_491 | ASW_2021_05_24_492 | |
---|---|---|---|---|---|---|---|---|---|---|---|---|---|---|---|---|---|---|---|---|---|
0 | 799.8442 | 6.517882e-02 | 6.611663e-02 | 6.766044e-02 | 7.767758e-02 | 8.016905e-02 | 8.028784e-02 | 0.079780 | 7.776978e-02 | 7.765637e-02 | ... | 0.048800 | 0.048767 | 0.048408 | 0.049178 | 0.047778 | 0.051763 | 0.052555 | 0.055020 | 0.056034 | 0.061171 |
1 | 800.3264 | 6.945459e-02 | 6.969134e-02 | 7.088943e-02 | 7.296595e-02 | 7.390441e-02 | 7.500930e-02 | 0.074624 | 7.745363e-02 | 7.897147e-02 | ... | 0.052965 | 0.053671 | 0.052923 | 0.054063 | 0.053984 | 0.055621 | 0.057292 | 0.059009 | 0.059942 | 0.066201 |
2 | 800.8085 | 7.292112e-02 | 7.288691e-02 | 7.361745e-02 | 6.737457e-02 | 6.700571e-02 | 6.841946e-02 | 0.068437 | 7.570509e-02 | 7.891863e-02 | ... | 0.056586 | 0.057301 | 0.056603 | 0.057588 | 0.059036 | 0.058832 | 0.060971 | 0.062315 | 0.063480 | 0.070444 |
3 | 801.2906 | 6.940653e-02 | 6.975350e-02 | 7.111548e-02 | 6.573625e-02 | 6.443463e-02 | 6.554944e-02 | 0.066081 | 7.037464e-02 | 7.083752e-02 | ... | 0.051400 | 0.051448 | 0.051381 | 0.052487 | 0.053773 | 0.053324 | 0.055295 | 0.055882 | 0.058406 | 0.063919 |
4 | 801.7727 | 6.659455e-02 | 6.678519e-02 | 6.828554e-02 | 6.814425e-02 | 6.737859e-02 | 6.831091e-02 | 0.068845 | 6.914572e-02 | 6.787662e-02 | ... | 0.046206 | 0.046325 | 0.046060 | 0.047885 | 0.049168 | 0.048616 | 0.049703 | 0.050537 | 0.053893 | 0.058902 |
... | ... | ... | ... | ... | ... | ... | ... | ... | ... | ... | ... | ... | ... | ... | ... | ... | ... | ... | ... | ... | ... |
6636 | 3998.2570 | 6.052594e-04 | 6.155879e-04 | 5.891753e-04 | 4.509248e-04 | 3.057191e-04 | 3.017703e-04 | 0.000373 | 3.303686e-04 | 5.509026e-04 | ... | 0.000525 | 0.000569 | 0.000513 | 0.000519 | 0.000521 | 0.000546 | 0.000569 | 0.000509 | 0.000523 | 0.000514 |
6637 | 3998.7390 | 3.875527e-04 | 3.993715e-04 | 3.591537e-04 | 2.900099e-04 | 1.944961e-04 | 1.994642e-04 | 0.000299 | 2.035522e-04 | 3.159683e-04 | ... | 0.000346 | 0.000352 | 0.000322 | 0.000330 | 0.000345 | 0.000352 | 0.000361 | 0.000334 | 0.000336 | 0.000338 |
6638 | 3999.2210 | -8.673617e-19 | -4.336809e-19 | -2.168404e-19 | -3.469447e-18 | -1.301043e-18 | 3.469447e-18 | 0.000121 | 2.168404e-18 | 1.734723e-18 | ... | 0.000000 | 0.000000 | 0.000000 | 0.000000 | 0.000000 | 0.000000 | 0.000000 | 0.000000 | 0.000000 | 0.000000 |
6639 | 3999.7030 | 7.357823e-04 | 7.429329e-04 | 7.613905e-04 | 3.697233e-04 | 6.489695e-05 | 1.846985e-05 | 0.000126 | 1.990229e-04 | 7.767737e-04 | ... | 0.000430 | 0.000447 | 0.000411 | 0.000422 | 0.000424 | 0.000413 | 0.000430 | 0.000414 | 0.000451 | 0.000435 |
6640 | 4000.1850 | 1.665731e-03 | 1.684217e-03 | 1.704210e-03 | 9.846366e-04 | 4.392129e-04 | 3.268367e-04 | 0.000441 | 5.654779e-04 | 1.681778e-03 | ... | 0.001176 | 0.001227 | 0.001154 | 0.001179 | 0.001158 | 0.001152 | 0.001200 | 0.001149 | 0.001240 | 0.001204 |
6641 rows Γ 493 columns
wavelength = DR2_Allscan_df.T.iloc[0]
wavelength
0 799.8442
1 800.3264
2 800.8085
3 801.2906
4 801.7727
...
6636 3998.2570
6637 3998.7390
6638 3999.2210
6639 3999.7030
6640 4000.1850
Name: Wavenumber, Length: 6641, dtype: float64
2.2 Function definitionΒΆ
def substraction(index1, date, index2):
# exp_date1 = input('date of experiment 1 (format YYYY_MM_DD) = ')
# index1 = int(input('index 1 = '))
# exp_date2 = input('date of experiment 2 (format YYYY_MM_DD) = ')
# index2 = int(input('index 2 = '))
data1 = DR2_Allscan_df
#data2 = pd.read_csv('D:\PhD-WS\Projects\PAC\{}}\DATA\{0}\Data\DR2_{}_All_data.csv'.format(XP,date))
data1 = data1.T.iloc[1:].T
#data2 = data2.T.iloc[1:].T
#Scan 1
list_name1 = list(data1.columns.values.tolist())
scan_place1 = 0
for i in range(len(list_name1)):
name_corr = list_name1[i].split('_')
if int(name_corr[-1]) == int(index1):
scan_place1 = i
scan1 = data1.T.iloc[scan_place1]
#Scan 2
list_name2 = list(data1.columns.values.tolist())
scan_place2 = 0
for i in range(len(list_name2)):
name_corr = list_name2[i].split('_')
if int(name_corr[-1]) == int(index2):
scan_place2 = i
scan2 = data1.T.iloc[scan_place2]
sub_scan = numpy.zeros(len(scan1))
for iscan in range(len(sub_scan)):
sub_scan[iscan] = scan2[iscan] - scan1[iscan]
return sub_scan
2.3 PlottingΒΆ
All RangeΒΆ
#date = "2020_09_15"
data1 = DR2_Allscan_df
data1 = data1.T.iloc[1:].T
#data = data.T.iloc[1:].T
#data = data1
nscan = len(list(data1.columns.values.tolist()))
print(nscan)
#print(data1)
fig = plt.figure(figsize=(16,16))
ax = fig.add_subplot()
ax.set_xlabel('Wavenumber (cm-1)')
ax.set_ylabel('Absorbance')
#ax.set_zlabel('Absorbance')
data_sub = []
data_sub_2 =[]
for iscan in range(nscan-1):
iscan = iscan+1
sub_scan = substraction(iscan, date, iscan+1)
df = pd.DataFrame({'Wavenumber': wavelength, "ASW_"+str(date)+"_" + str(iscan) +"-" + str(iscan+1): sub_scan})
#df.to_csv('D:\PhD-WS\Projects\PAC\XP_1-1\DATA\{}\Data\DP1\DP1_{}_sub-scan_{}.csv'.format(date,date, iscan))
#data_int.append(df)
data_sub.append(
{
'Name' : "ASW_"+str(date)+"_" + str(iscan) +"-" + str(iscan+1),
'Wavenumber' : wavelength,
'sub_scan' : sub_scan,
})
data_sub_2.append(df)
plt.plot(wavelength, sub_scan, c=cm.jet(iscan/nscan), label = "scan{0}".format(str(iscan)))
plt.title('{}_Substraction scans'.format(date), pad=20).set_fontsize(18)
ax.hlines(0,3900,2900)
plt.xlim([3800,2900])
plt.legend()
plt.show()
492
C:\Users\vmrd3\Anaconda3\lib\site-packages\IPython\core\pylabtools.py:132: UserWarning: Creating legend with loc="best" can be slow with large amounts of data.
fig.canvas.print_figure(bytes_io, **kw)

Range 1ΒΆ
Insert condition to use only items present in range 1
#date = "2020_09_15"
data1 = DR2_Allscan_df
data1 = data1.T.iloc[1:].T
#data = data.T.iloc[1:].T
#data = data1
nscan = len(list(data1.columns.values.tolist()))
print(nscan)
#print(data1)
fig = plt.figure(figsize=(10,10))
ax = fig.add_subplot()
ax.set_xlabel('Wavenumber (cm-1)')
ax.set_ylabel('Absorbance')
#ax.set_zlabel('Absorbance')
data_sub = []
data_sub_2 =[]
for iscan in file_number1:
iscan = iscan+1
sub_scan = substraction(iscan, date, iscan+1)
df = pd.DataFrame({'Wavenumber': wavelength, "ASW_"+str(date)+"_" + str(iscan) +"-" + str(iscan+1): sub_scan})
#df.to_csv('D:\PhD-WS\Projects\PAC\XP_1-1\DATA\{}\Data\DP1\DP1_{}_sub-scan_{}.csv'.format(date,date, iscan))
#data_int.append(df)
data_sub.append(
{
'Name' : "ASW_"+str(date)+"_" + str(iscan) +"-" + str(iscan+1),
'Wavenumber' : wavelength,
'sub_scan' : sub_scan,
})
data_sub_2.append(df)
plt.plot(wavelength, sub_scan, c=cm.jet(iscan/nscan), label = "scan{0}".format(str(iscan)))
plt.title('{}_Substraction scans'.format(date), pad=20).set_fontsize(18)
ax.hlines(0,3900,2900)
plt.xlim([3800,2900])
plt.legend()
plt.show()
492
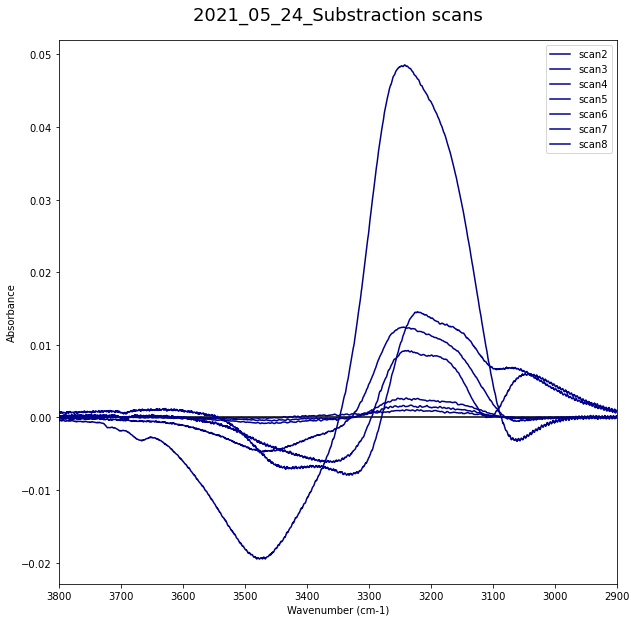
df
Wavenumber | ASW_2021_05_24_8-9 | |
---|---|---|
0 | 799.8442 | -1.134107e-04 |
1 | 800.3264 | 1.517846e-03 |
2 | 800.8085 | 3.213542e-03 |
3 | 801.2906 | 4.628880e-04 |
4 | 801.7727 | -1.269106e-03 |
... | ... | ... |
6636 | 3998.2570 | 2.205339e-04 |
6637 | 3998.7390 | 1.124161e-04 |
6638 | 3999.2210 | -4.336809e-19 |
6639 | 3999.7030 | 5.777508e-04 |
6640 | 4000.1850 | 1.116301e-03 |
6641 rows Γ 2 columns
data_sub
[{'Name': 'ASW_2021_05_24_2-3',
'Wavenumber': 0 799.8442
1 800.3264
2 800.8085
3 801.2906
4 801.7727
...
6636 3998.2570
6637 3998.7390
6638 3999.2210
6639 3999.7030
6640 4000.1850
Name: Wavenumber, Length: 6641, dtype: float64,
'sub_scan': array([1.54380954e-03, 1.19808933e-03, 7.30539117e-04, ...,
2.16840434e-19, 1.84575997e-05, 1.99932794e-05])},
{'Name': 'ASW_2021_05_24_3-4',
'Wavenumber': 0 799.8442
1 800.3264
2 800.8085
3 801.2906
4 801.7727
...
6636 3998.2570
6637 3998.7390
6638 3999.2210
6639 3999.7030
6640 4000.1850
Name: Wavenumber, Length: 6641, dtype: float64,
'sub_scan': array([ 1.00171360e-02, 2.07651706e-03, -6.24288189e-03, ...,
-3.25260652e-18, -3.91667293e-04, -7.19573885e-04])},
{'Name': 'ASW_2021_05_24_4-5',
'Wavenumber': 0 799.8442
1 800.3264
2 800.8085
3 801.2906
4 801.7727
...
6636 3998.2570
6637 3998.7390
6638 3999.2210
6639 3999.7030
6640 4000.1850
Name: Wavenumber, Length: 6641, dtype: float64,
'sub_scan': array([ 2.49147249e-03, 9.38463666e-04, -3.68855160e-04, ...,
2.16840434e-18, -3.04826309e-04, -5.45423718e-04])},
{'Name': 'ASW_2021_05_24_5-6',
'Wavenumber': 0 799.8442
1 800.3264
2 800.8085
3 801.2906
4 801.7727
...
6636 3998.2570
6637 3998.7390
6638 3999.2210
6639 3999.7030
6640 4000.1850
Name: Wavenumber, Length: 6641, dtype: float64,
'sub_scan': array([ 1.18793290e-04, 1.10489031e-03, 1.41374733e-03, ...,
4.77048956e-18, -4.64270998e-05, -1.12376200e-04])},
{'Name': 'ASW_2021_05_24_6-7',
'Wavenumber': 0 799.8442
1 800.3264
2 800.8085
3 801.2906
4 801.7727
...
6636 3998.2570
6637 3998.7390
6638 3999.2210
6639 3999.7030
6640 4000.1850
Name: Wavenumber, Length: 6641, dtype: float64,
'sub_scan': array([-5.07472153e-04, -3.85217634e-04, 1.70768859e-05, ...,
1.20905107e-04, 1.07518578e-04, 1.13815049e-04])},
{'Name': 'ASW_2021_05_24_7-8',
'Wavenumber': 0 799.8442
1 800.3264
2 800.8085
3 801.2906
4 801.7727
...
6636 3998.2570
6637 3998.7390
6638 3999.2210
6639 3999.7030
6640 4000.1850
Name: Wavenumber, Length: 6641, dtype: float64,
'sub_scan': array([-2.01059091e-03, 2.82954489e-03, 7.26855070e-03, ...,
-1.20905107e-04, 7.30345003e-05, 1.24826107e-04])},
{'Name': 'ASW_2021_05_24_8-9',
'Wavenumber': 0 799.8442
1 800.3264
2 800.8085
3 801.2906
4 801.7727
...
6636 3998.2570
6637 3998.7390
6638 3999.2210
6639 3999.7030
6640 4000.1850
Name: Wavenumber, Length: 6641, dtype: float64,
'sub_scan': array([-1.13410710e-04, 1.51784552e-03, 3.21354176e-03, ...,
-4.33680869e-19, 5.77750781e-04, 1.11630056e-03])}]
data_sub_2
[ Wavenumber ASW_2021_05_24_2-3
0 799.8442 1.543810e-03
1 800.3264 1.198089e-03
2 800.8085 7.305391e-04
3 801.2906 1.361979e-03
4 801.7727 1.500349e-03
... ... ...
6636 3998.2570 -2.641260e-05
6637 3998.7390 -4.021780e-05
6638 3999.2210 2.168404e-19
6639 3999.7030 1.845760e-05
6640 4000.1850 1.999328e-05
[6641 rows x 2 columns],
Wavenumber ASW_2021_05_24_3-4
0 799.8442 1.001714e-02
1 800.3264 2.076517e-03
2 800.8085 -6.242882e-03
3 801.2906 -5.379231e-03
4 801.7727 -1.412898e-04
... ... ...
6636 3998.2570 -1.382505e-04
6637 3998.7390 -6.914371e-05
6638 3999.2210 -3.252607e-18
6639 3999.7030 -3.916673e-04
6640 4000.1850 -7.195739e-04
[6641 rows x 2 columns],
Wavenumber ASW_2021_05_24_4-5
0 799.8442 2.491472e-03
1 800.3264 9.384637e-04
2 800.8085 -3.688552e-04
3 801.2906 -1.301614e-03
4 801.7727 -7.656528e-04
... ... ...
6636 3998.2570 -1.452057e-04
6637 3998.7390 -9.551389e-05
6638 3999.2210 2.168404e-18
6639 3999.7030 -3.048263e-04
6640 4000.1850 -5.454237e-04
[6641 rows x 2 columns],
Wavenumber ASW_2021_05_24_5-6
0 799.8442 1.187933e-04
1 800.3264 1.104890e-03
2 800.8085 1.413747e-03
3 801.2906 1.114804e-03
4 801.7727 9.323114e-04
... ... ...
6636 3998.2570 -3.948800e-06
6637 3998.7390 4.968100e-06
6638 3999.2210 4.770490e-18
6639 3999.7030 -4.642710e-05
6640 4000.1850 -1.123762e-04
[6641 rows x 2 columns],
Wavenumber ASW_2021_05_24_6-7
0 799.8442 -0.000507
1 800.3264 -0.000385
2 800.8085 0.000017
3 801.2906 0.000531
4 801.7727 0.000534
... ... ...
6636 3998.2570 0.000071
6637 3998.7390 0.000099
6638 3999.2210 0.000121
6639 3999.7030 0.000108
6640 4000.1850 0.000114
[6641 rows x 2 columns],
Wavenumber ASW_2021_05_24_7-8
0 799.8442 -0.002011
1 800.3264 0.002830
2 800.8085 0.007269
3 801.2906 0.004294
4 801.7727 0.000301
... ... ...
6636 3998.2570 -0.000042
6637 3998.7390 -0.000095
6638 3999.2210 -0.000121
6639 3999.7030 0.000073
6640 4000.1850 0.000125
[6641 rows x 2 columns],
Wavenumber ASW_2021_05_24_8-9
0 799.8442 -1.134107e-04
1 800.3264 1.517846e-03
2 800.8085 3.213542e-03
3 801.2906 4.628880e-04
4 801.7727 -1.269106e-03
... ... ...
6636 3998.2570 2.205339e-04
6637 3998.7390 1.124161e-04
6638 3999.2210 -4.336809e-19
6639 3999.7030 5.777508e-04
6640 4000.1850 1.116301e-03
[6641 rows x 2 columns]]
Range 2ΒΆ
#date = "2020_09_15"
data1 = DR2_Allscan_df
data1 = data1.T.iloc[1:].T
#data = data.T.iloc[1:].T
#data = data1
nscan = len(list(data1.columns.values.tolist()))
print(nscan)
#print(data1)
fig = plt.figure(figsize=(10,10))
ax = fig.add_subplot()
ax.set_xlabel('Wavenumber (cm-1)')
ax.set_ylabel('Absorbance')
#ax.set_zlabel('Absorbance')
data_sub = []
data_sub_2 =[]
for iscan in file_number2:
iscan = iscan+1
sub_scan = substraction(iscan, date, iscan+1)
df = pd.DataFrame({'Wavenumber': wavelength, "ASW_"+str(date)+"_" + str(iscan) +"-" + str(iscan+1): sub_scan})
#df.to_csv('D:\PhD-WS\Projects\PAC\XP_1-1\DATA\{}\Data\DP1\DP1_{}_sub-scan_{}.csv'.format(date,date, iscan))
#data_int.append(df)
data_sub.append(
{
'Name' : "ASW_"+str(date)+"_" + str(iscan) +"-" + str(iscan+1),
'Wavenumber' : wavelength,
'sub_scan' : sub_scan,
})
data_sub_2.append(df)
plt.plot(wavelength, sub_scan, c=cm.jet(iscan/nscan), label = "scan{0}".format(str(iscan)))
plt.title('{}_Substraction scans'.format(date), pad=20).set_fontsize(18)
ax.hlines(0,3900,2900)
plt.xlim([3800,2900])
plt.legend()
plt.show()
31
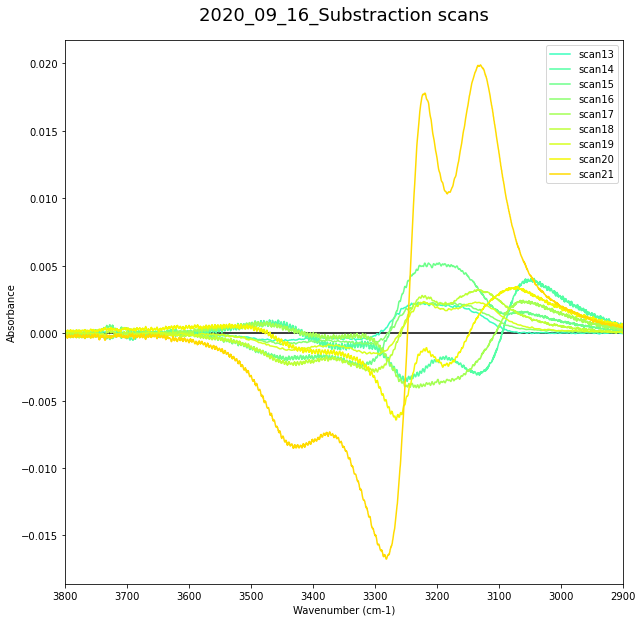
Range 3ΒΆ
#date = "2020_09_15"
data1 = DR2_Allscan_df
data1 = data1.T.iloc[1:].T
#data = data.T.iloc[1:].T
#data = data1
nscan = len(list(data1.columns.values.tolist()))
print(nscan)
#print(data1)
fig = plt.figure(figsize=(10,10))
ax = fig.add_subplot()
ax.set_xlabel('Wavenumber (cm-1)')
ax.set_ylabel('Absorbance')
#ax.set_zlabel('Absorbance')
data_sub = []
data_sub_2 =[]
for iscan in file_number3:
iscan = iscan+1
sub_scan = substraction(iscan, date, iscan+1)
df = pd.DataFrame({'Wavenumber': wavelength, "ASW_"+str(date)+"_" + str(iscan) +"-" + str(iscan+1): sub_scan})
#df.to_csv('D:\PhD-WS\Projects\PAC\XP_1-1\DATA\{}\Data\DP1\DP1_{}_sub-scan_{}.csv'.format(date,date, iscan))
#data_int.append(df)
data_sub.append(
{
'Name' : "ASW_"+str(date)+"_" + str(iscan) +"-" + str(iscan+1),
'Wavenumber' : wavelength,
'sub_scan' : sub_scan,
})
data_sub_2.append(df)
plt.plot(wavelength, sub_scan, c=cm.jet(iscan/nscan), label = "scan{0}".format(str(iscan)))
plt.title('{}_Substraction scans'.format(date), pad=20).set_fontsize(18)
ax.hlines(0,3900,2900)
plt.xlim([3800,2900])
plt.ylim([-0.0075,0.0075])
#plt.legend()
plt.show()
492
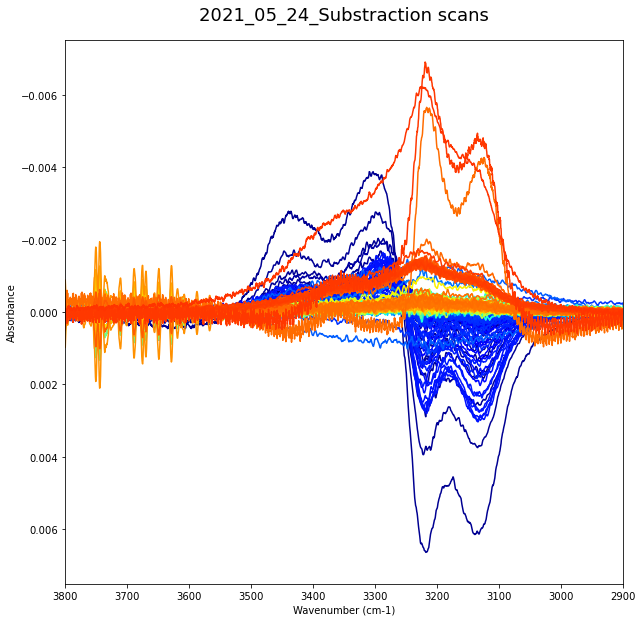
Range 4ΒΆ
#date = "2020_09_15"
data1 = DR2_Allscan_df
data1 = data1.T.iloc[1:].T
#data = data.T.iloc[1:].T
#data = data1
nscan = len(list(data1.columns.values.tolist()))
print(nscan)
#print(data1)
fig = plt.figure(figsize=(10,10))
ax = fig.add_subplot()
ax.set_xlabel('Wavenumber (cm-1)')
ax.set_ylabel('Absorbance')
#ax.set_zlabel('Absorbance')
data_sub = []
data_sub_2 =[]
for iscan in file_number4:
iscan = iscan+1
sub_scan = substraction(iscan, date, iscan+1)
df = pd.DataFrame({'Wavenumber': wavelength, "ASW_"+str(date)+"_" + str(iscan) +"-" + str(iscan+1): sub_scan})
#df.to_csv('D:\PhD-WS\Projects\PAC\XP_1-1\DATA\{}\Data\DP1\DP1_{}_sub-scan_{}.csv'.format(date,date, iscan))
#data_int.append(df)
data_sub.append(
{
'Name' : "ASW_"+str(date)+"_" + str(iscan) +"-" + str(iscan+1),
'Wavenumber' : wavelength,
'sub_scan' : sub_scan,
})
data_sub_2.append(df)
plt.plot(wavelength, sub_scan, c=cm.jet(iscan/nscan), label = "scan{0}".format(str(iscan)))
plt.title('{}_Substraction scans'.format(date), pad=20).set_fontsize(18)
ax.hlines(0,3900,2900)
plt.xlim([3800,2900])
plt.legend()
plt.show()
492
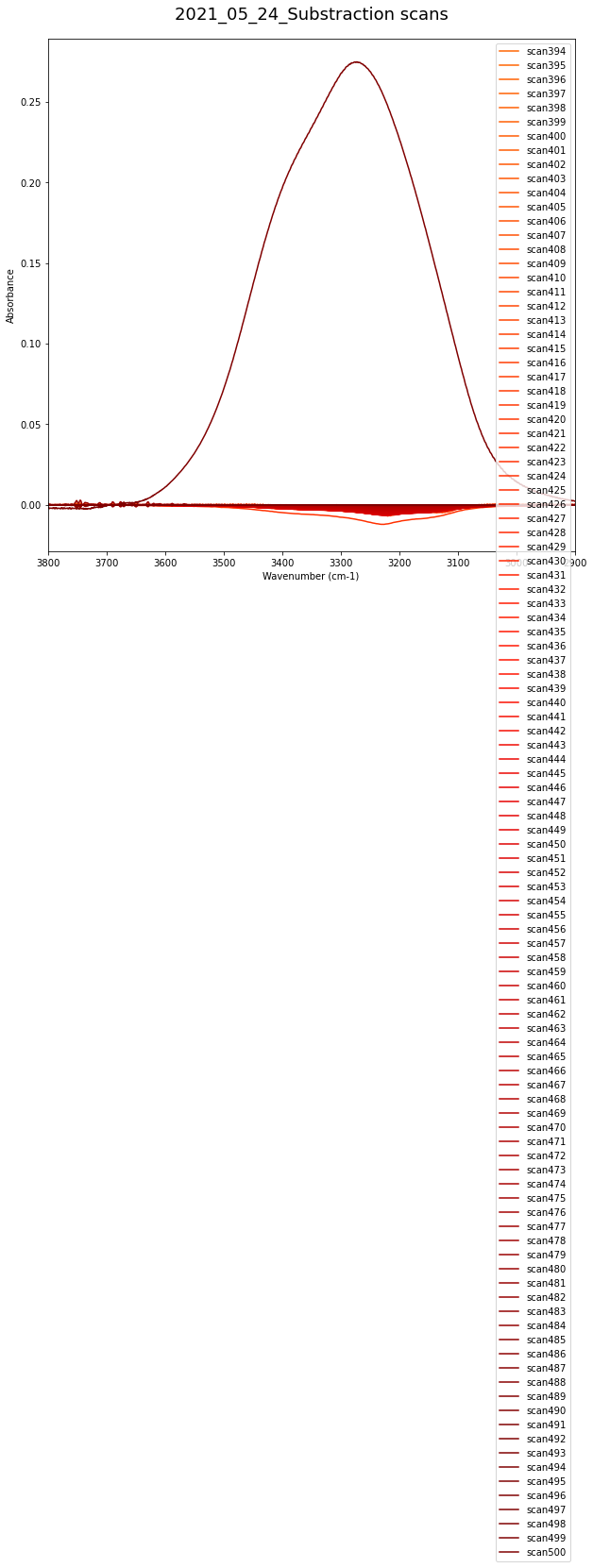
Preliminary analysisΒΆ
Purpose here would be (for the different physical processes that the ice undergo upon annealing) to investigate the different contributions lost and gain from the substraction spectra
This will subsequently be used as initial guesses for the gaussian fit of the whole samples
An intermediate gaussian fitting routine could be implemented here with the substraction spectra
Export Data Sub as csvΒΆ
ConcatenationΒΆ
data_sub
data_sub_2_df = reduce(lambda left,right: pd.merge(left,right, on=['Wavenumber'],how='outer'), data_sub_2)
Export as csvΒΆ
\(\color{green}{\text{DP3 : Gaussian Fitting}}\) ΒΆ
Gaussian fit 1ΒΆ
x = DR2_A_df["Wavenumber"]
y = DR2_A_df["ASW_2020_09_16_1"]
pars = exp_mod.guess(y, x=x)
gauss1 = GaussianModel(prefix='g1_')
pars.update(gauss1.make_params())
pars['g1_center'].set(value=3400, min=3300, max=3500)
pars['g1_sigma'].set(value=15, min=3)
pars['g1_amplitude'].set(value=10, min=1)
gauss2 = GaussianModel(prefix='g2_')
pars.update(gauss2.make_params())
pars['g2_center'].set(value=3260, min=3200, max=3300)
pars['g2_sigma'].set(value=15, min=3)
pars['g2_amplitude'].set(value=10, min=1)
mod = gauss1 + gauss2
init = mod.eval(pars, x=x)
out = mod.fit(y, pars, x=x)
print(out.fit_report(min_correl=0.5))
fig, axes = plt.subplots(1, 2, figsize=(12.8, 4.8))
axes[0].plot(x, y, 'b')
axes[0].plot(x, init, 'k--', label='initial fit')
axes[0].plot(x, out.best_fit, 'r-', label='best fit')
axes[0].invert_xaxis()
axes[0].legend(loc='best')
comps = out.eval_components(x=x)
axes[1].plot(x, y, 'b')
axes[1].plot(x, comps['g1_'], 'g--', label='Gaussian component 1')
axes[1].plot(x, comps['g2_'], 'm--', label='Gaussian component 2')
axes[1].invert_xaxis()
axes[1].legend(loc='best')
plt.show()
# <end examples/doc_builtinmodels_nistgauss.py>
---------------------------------------------------------------------------
NameError Traceback (most recent call last)
<ipython-input-42-82c111bdc377> in <module>
----> 1 x = DR2_A_df["Wavenumber"]
2 y = DR2_A_df["ASW_2020_09_16_1"]
3
4 pars = exp_mod.guess(y, x=x)
5
NameError: name 'DR2_A_df' is not defined